What is a Columnar Database?
Columnar databases are purpose-built for fast, scalable, dimensional analysis.
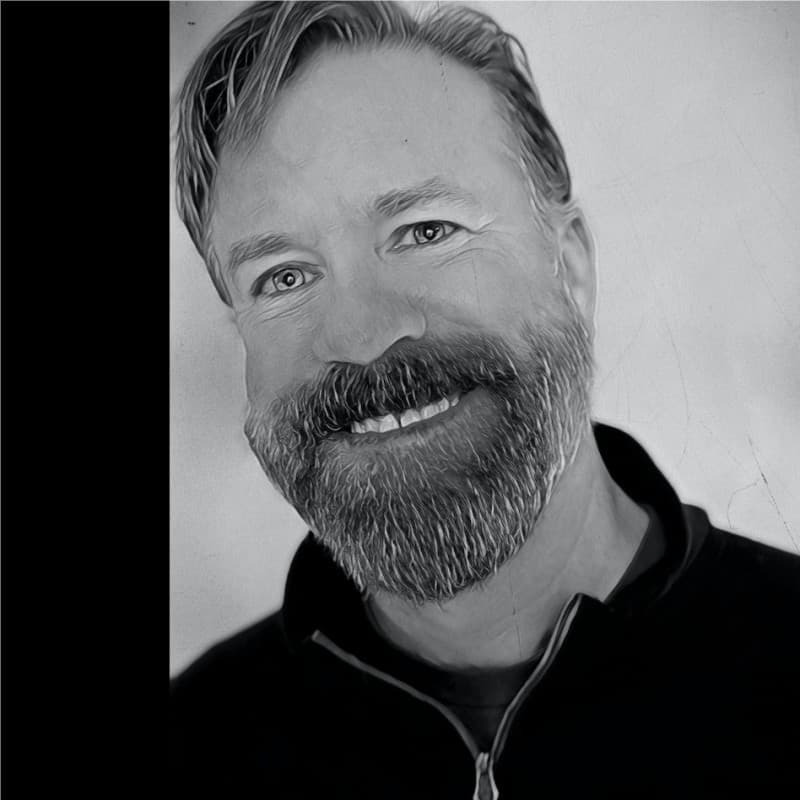
Columnar databases are the standard for analytic databases, particularly when performance, scalability, and query speed really matter. Whether you’re powering business dashboards, anomaly detection, or customer-facing data products, a columnar database can give you the low-latency querying you need—even on massive datasets.
In this post, we’ll break down what a columnar database is and what makes column-oriented storage so important for real-time analytics at scale.
Column-oriented vs Row-oriented Databases
A columnar database (or column-oriented database) stores data by columns rather than by rows. That might sound like a small change, but it has a big impact—especially for analytical queries that need to scan and aggregate large volumes of data quickly.
Let’s compare:
In a row-based database (like PostgreSQL or MySQL):
Data is stored like this:
ID | Name | Age | Country |
1 | Tom | 28 | US |
2 | Ana | 31 | UK |
3 | Raj | 25 | IN |
All values in a row are stored together on disk. This is great for transactional workloads (OLTP), where you’re often reading or writing full records individually.
In a column-based database:
The same data is stored like this:
- ID: 1, 2, 3
- Name: “Tom”, “Ana”, “Raj”
- Age: 28, 31, 25
- Country: “US”, “UK”, “IN”
Each column is stored separately and contiguously.
This structure is incredibly efficient for analytical queries—especially those that aggregate or filter on just a few columns out of a wide dataset.
Why is columnar storage faster for analytics?
Let’s walk through a typical use case: you want to calculate the average order value for users in the last 24 hours, grouped by country.
To answer this, your query only needs to scan a few specific columns:
- timestamp
- country
- order_value
A row based database has to load every column in the table—even those you don’t need, like user name, email, or address. That results in unnecessary I/O, higher memory usage, and slower performance.
Columnar databases take a different approach. Because each column is stored separately, only the relevant columns are scanned for a given query. This leads to:
- Lower disk I/O
- Faster query execution
- Smaller memory footprint
The benefits become even more pronounced in wide tables with hundreds or thousands of columns—where the ability to scan just a handful of fields makes a massive difference.
Columnar storage can be further optimized through features that maximize query efficiency:
- Fast scans: Only the queried columns are read from disk
- Sorted Columns: Like data is grouped next to each other, which makes it quicker for the database to extract the rows it needs from a segment of data
- Efficient compression: Similar values within columns compress extremely well
- Smart indexing: Advanced columnar databases such as Apache Pinot build specialized indexes—like inverted, star-tree, and sorted indexes—on a per-column basis, enabling lightning-fast filtering and aggregations
How many columns is too many?
One of the advantages of a columnar database is that it scales well with wide tables—meaning tables with hundreds or even thousands of columns.
In practice, the number of columns can vary widely:
- Narrow tables (10–50 columns) are common for focused use cases like time-series metrics or real-time monitoring dashboards.
- Wide tables (500–1,000+ columns) are increasingly common in modern analytics environments, especially in use cases like customer 360 views, product analytics, ad tech, observability, or telemetry data—where each column represents a unique dimension or attribute.
The more columns in your dataset (especially wide tables), the bigger the performance benefit.
Updates (and writes) with Columnar Data
Columnar databases are traditionally optimized for read-heavy, analytical workloads, which often means they’re not well-suited for high-frequency writes or real-time updates. Many conventional columnar systems—especially those designed for batch-oriented data warehousing—handle incoming data by inserting new records in batches, making them less effective when dealing with mutable datasets that require frequent updates.
To address this, some modern columnar databases have introduced architectural approaches that support real-time upserts while preserving query performance. Instead of performing costly in-place updates, these systems treat all incoming records—whether new or updated—as inserts. When an update is detected, the older version of the record is marked as inactive, ensuring it doesn’t appear in future queries. This approach avoids duplicate results while maintaining consistency.
In the background, lightweight cleanup processes periodically remove deactivated records to maintain storage efficiency without impacting query speed or freshness.
This model allows for fresh, real-time data to remain queryable with low latency—even in high-ingestion environments—a capability that remains relatively rare among traditional columnar databases, but increasingly essential in modern analytics use cases.
How to Scale a Columnar Database for Analytics
When evaluating a columnar database for real-time analytics, scalability is essential. But it’s important to distinguish between two critical dimensions of scale: scaling for data volume and scaling for query concurrency. Many systems are optimized for one, but few excel at both.
Scaling for Data Volume
Most modern columnar databases are relatively comparable when it comes to handling large volumes of data. They use well-established techniques like distributed processing, sharding, and columnar compression to efficiently manage massive datasets. These systems typically scale horizontally, allowing organizations to process billions of rows and store petabytes of data across clusters. With parallel query execution, they can support complex aggregations and scans with minimal latency, even over wide, high-cardinality datasets.
Scaling for Concurrency
Where differences start to emerge is in query concurrency. While many columnar systems handle data volume well, they often struggle when query demand grows—especially in multi-tenant or customer-facing environments where hundreds or thousands of users may query the system simultaneously. In these cases, it’s common to require additional clusters or replicated instances to support growing user groups, which increases operational complexity and cost.
Some newer systems are purpose-built to address this challenge, with architectures optimized for high QPS (queries per second) and native support for multi-tenant workloads. These platforms allow multiple teams or applications to share infrastructure while maintaining isolation, consistency, and performance—making them well-suited for organizations that need to scale both their data and their users, without trade-offs.
Examples of Popular Columnar Databases
Columnar databases are well-suited for batch-oriented data warehousing workloads, offering efficient storage and fast execution of large-scale aggregations used in historical reporting and business intelligence. Their architecture allows for high compression and parallel processing, making them ideal for running complex queries over massive datasets.
Columnar databases are also increasingly used for real-time dimensional analysis as they enable sub-second filtering, grouping, and aggregations across high-cardinality, fast-changing data. This makes them a powerful choice for modern applications that require low-latency insights and operational visibility.
Here are some of the most widely used:
Popular Column Oriented Databases as Data Warehouses
- Snowflake – A proprietary, cloud-native data warehouse known for its separation of storage and compute. Snowflake is a popular choice for data warehousing and BI workloads due to its multi-cloud availability, offering deployment across AWS, Azure, and Google Cloud—unlike other major solutions that are tied to a single cloud provider.
Cons: Snowflake costs can rise quickly at scale or under unpredictable usage patterns.
- Amazon Redshift – A proprietary, fully managed data warehouse service offered by AWS, designed for large-scale, SQL-based analytics. It integrates tightly with the AWS ecosystem and is commonly used for traditional batch-oriented data warehousing and business intelligence workloads. As an AWS product, vendor lock in is an issue.
Cons: Redshift’s performance can decline with concurrent query load, and it often requires manual tuning and optimization to maintain responsiveness at scale.
- Google BigQuery – A proprietary, serverless data warehouse developed by Google Cloud, built for interactive SQL analytics at scale. It is tightly integrated with Google’s cloud services and is optimized for high-throughput batch queries and machine learning use cases.
Cons: BigQuery operates on a pay-per-query model, which can lead to unpredictable costs, especially for exploratory or ad hoc workloads. It also has latency limitations for real-time use cases and may not be the best fit for low-latency, high-concurrency applications. As a Google Cloud product, vendor lock in is an issue.
Popular Column Oriented Databases for Real-Time Dimensional Analysis
- Apache Pinot – An open-source, real-time distributed OLAP database built for subsecond analytics on high-throughput, high-cardinality event data. Pinot is uniquely capable of handling real-time upserts and mutable datasets, making it well-suited for applications that require fresh, accurate data—such as customer-facing analytics, personalization engines, and operational intelligence. Pinot is designed to support hundreds of thousands of concurrent users at petabyte scale, and is often adopted by organizations with many real-time use cases looking for a unified analytics platform.
Cons: As a multi-tenant platform, provisioning and managing Pinot at scale can be complex, particularly for teams without prior distributed systems experience—though managed service providers like StarTree offer fully hosted solutions to reduce operational overhead.
- ClickHouse – An open-source, high-performance OLAP database optimized for fast queries on large, denormalized tables. ClickHouse shines in scenarios like web analytics where ultra-fast aggregations and filtering are critical, the data is immutable, and the data model is simple. While technically not real-time in the strictest sense—it processes data in microbatches—ClickHouse is included here because its query performance sets it apart from traditional data warehouses.
Cons: Despite its speed, ClickHouse is not optimized for mutable datasets or frequent upserts, making it less suitable for applications that require real-time consistency or row-level updates. Performance can degrade as the number of simultaneous users or queries increases, making it less ideal for high-concurrency, user-facing applications
- Apache Druid – An open-source analytics database designed for low-latency queries over both streaming and batch data. Druid is widely used for interactive dashboards, operational monitoring, and scenarios where fast ingestion and time-based filtering are essential. Its native support for approximate algorithms and flexible ingestion model make it a strong choice for exploratory analytics and alerting use cases.
Cons: Druid tends to struggle with mutable data and upserts, making it less ideal for workloads that require frequent updates or corrections to existing records. Druid also is best for a small number of users as performance degrades with concurrency.
Each of these systems brings different strengths depending on the specific requirements around query latency, data latency, concurrency, cost, and scale. Choosing the right columnar database often depends on whether your priority is historical analysis, real-time insight, or a combination of both.
In sum.
Columnar Database | Row-oriented Database |
✅ High performance for analytical queries | ❌ Inefficient for analytical queries |
✅ Efficient storage and compression | ❌ Suboptimal storage and compression |
✅ Ease of scalability | ❌ Complex and expensive to scale |
❌ Slower for transactional workloads | ✅ Efficient for transactional workloads |
❌ Low Concurrency ** | ✅ High Concurrency |
❌ Higher overhead for updates and inserts ** | ✅ Easier modification of data |
** Apache Pinot is exceptional amongst column-oriented databases in that it utilizes advanced indexing strategies to support high concurrency. This makes it ideal for providing summary data for high-traffic applications and dashboards with lots of users and requests per second. Discover how specialized indexes in Apache Pinot enable responsive queries under high load.
One of the easiest ways to get started with Apache Pinot is with StarTree Cloud. Book a meeting with a Product Expert Today