The Power of Automated Anomaly Detection for Rideshare Booking Metrics
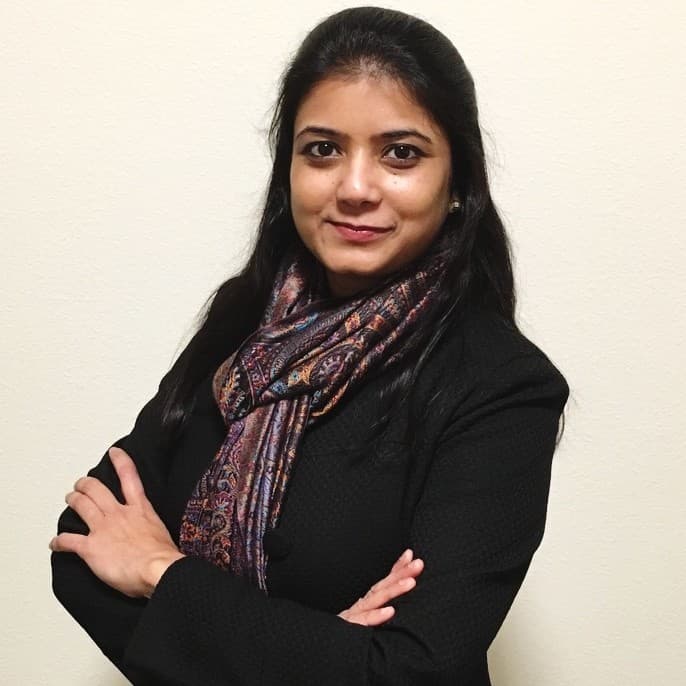
Introduction
As the rideshare industry grows, monitoring and analyzing booking metrics has become increasingly important for companies to stay competitive and provide a satisfactory customer experience. Automated Anomaly Detection and anomaly detection tools can help rideshare companies avoid potential issues and deliver a better customer experience overall.
The Benefits of Automated Anomaly Detection
Automated Anomaly Detection is a process that involves automatically collecting and analyzing data from various sources to identify trends and anomalies. Regarding rideshare booking metrics, this can include pickup and drop-off times, driver ratings, and customer feedback. By using Automated Anomaly Detection, rideshare companies can quickly identify trends that could impact customer satisfaction, such as long wait times or a surge in demand that could lead to a shortage of available drivers.
Automated Anomaly Detection also allows rideshare companies to address these issues before they impact their customers. Issues can include sudden spikes or drops in bookings or changes in customer behavior, like a sudden increase in cancellations or a decrease in driver ratings.
For example, suppose a company notices a trend of long wait times at a particular location. In that case, they can allocate more drivers to that area in advance to ensure customer pick-up promptly. By addressing issues proactively, rideshare companies can improve customer satisfaction and reduce the likelihood of negative reviews or complaints.
Anomaly detection can also help rideshare companies identify potential fraud or security issues. For example, if a driver suddenly starts consistently taking longer routes or making unusual stops, this could be a sign of fraud or unsafe behavior. By identifying these issues early, rideshare companies can take action to protect their customers and ensure that their experiences remain positive.
What is the solution?
StarTree ThirdEye can help automate rideshare booking metrics monitoring to achieve optimal customer satisfaction.
What is StarTree ThirdEye, and Why use it?
StarTree ThirdEye fast-tracks problem-solving with applied real-time anomaly detection on time series data and interactive root-cause analysis to unlock actionable insights!
Benefits of using StarTree ThirdEye
Reduced Time for Detection
StarTree ThirdEye can easily connect to real-time and historical data sources at scale and detect anomalies with a point-and-click experience. Allows monitoring of multiple time series in a given dataset in real-time.
Allows to run aggregated queries such as Sum/Avg/Count and simple/advanced statistical methods to detect outliers at scale in sub-second response time.
Applied Science
It runs outlier detection with applied science (out-of-the-box Anomaly Detection models) on time series data. Allows users to fine-tune these detection models for reduced false alarms with easy-to-use configurations based on the business context. Users don’t need to write algorithms or maintain models and data pipelines to detect outliers.
Reduced Time to Resolution
An interactive UI performs root cause analysis in case of an incident. It unlocks actionable insights such as top contributors and heatmap of dimensions contributing to the spike or drop in the critical metrics in an easy-to-use interface.
It allows performing dimension drills at scale in sub-second response time, helping with faster time to unlock actionable insights.
Intuitive User Interface, Customization, and Flexibility
It has a user-friendly interface that makes it easy to set up and use with a point-and-click-low code/no-code experience.
Users can customize the algorithms and parameters and build custom apps using APIs to solve custom business use cases.
Support and Documentation
StarTree ThirdEye comes with comprehensive documentation and support from StarTree in our community Slack.
How to automate monitoring rideshare booking metrics to improve customer satisfaction
Step 1: Prepare and onboard rideshare booking data and ingest it to Apache Pinot™
Download this Sample Data and upload it to Apache Pinot. This sample dataset contains rideshare time series data with ride cancellations, rides completed, ride request wait time, and API failures while booking a ride. If you are a StarTree Cloud user or StarTree Free Trial user, you can use Data Manager with a point-and-click experience to ingest data. For StarTree ThirdEye Community users or Apache Pinot OSS users, please refer to these recipes to ingest data to a local Pinot instance.
Here is a screenshot of the sample dataset.
Step-2: Create and configure Automated Anomaly Detection alerts in StarTree ThirdEye
Once the data is loaded and onboarded, log in to ThirdEye and configure alerts to detect outliers using a guided onboarding flow. This onboarding will teach you to configure and create alerts to automate monitoring and outlier detection in rideshare booking KPIs/metrics.
Here are the links one can leverage to get started using ThirdEye:
To create alerts, inspect the time series for trends, seasonality, and obvious outliers. ThirdEye’s alert preview lets us view the time series and the predicted anomalies. Preview the data using the simplest alert template, the startree-threshold.
You can see a fairly cyclic pattern each day with some variation between the days. There is a large outlier towards the end of March. The cyclic pattern is called seasonality. All our key metrics, wait time, cancellations, and API errors show a seasonality period of 1 day. At the beginning of the day, the metrics are fairly low. They reach a peak towards the middle of the day and end at roughly the same value as the morning.
A simple threshold alert will not work for this data since the threshold would depend on the time of day, so ordinary monitoring/alerting tools are insufficient. If you want to monitor different data dimensions, it would require time-dependent thresholds. Instead, you can use the startree-ets-dx template to model data with seasonality and trends.
Step-3: Result and anomaly investigation
Once the alerts are configured, they run at a certain cadence to detect anomalies. The wait time is monitored per city and ride type in the following example. The outliers are detected at scheduled intervals and notified via email/slack/webhook in case there is a spike or drop based on the seasonality and historical data.
Once an outlier is detected, you can further investigate the data by looking at the heatmap and performing dimension drills to understand which dimensions are the top contributors to the deviation. StarTree ThirdEye provides a “Top contributors” view summarizing the deviation’s top contributors.
In this example, the blue indicates the wait time spikes, and the red indicates drops. The spikes or drops can be healthy or unhealthy based on context. In this case, the spike is unhealthy. Users can further drill down on the wait time dimension to see where the spike comes from.
After the drill-down, most spikes are in San Francisco, shared ride-type, on mobile, Andriod version RS200. As a corrective action, app engineers can detect this as soon as it occurs, identify any software defect causing this sudden spike or drop, and take necessary action accordingly.
Conclusion
Automated Anomaly Detection and anomaly detection are powerful tools that can help rideshare companies improve customer satisfaction by identifying potential issues before they impact their customers.
By using these tools, rideshare companies can stay ahead of the competition and deliver a better experience overall. As the rideshare industry continues to grow and evolve, it will be increasingly important for companies to invest in these technologies to ensure they remain competitive and provide the best possible service for their customers.
If you want to explore and try StarTree ThirdEye, you can:
- Request a demo
- Sign-up for StarTree Free Trial. No credit card is required to get started. Learn More.
- Join the community
- Learn more about StarTree ThirdEye
Team credits
StarTree ThirdEye team – Tushar Thole, Suvodeep Payne, Cyril de Catheu, Shounak Kulkarni, Steven Pham, Aakarsh Chopra.
Marketing Team – Allison Murphy.